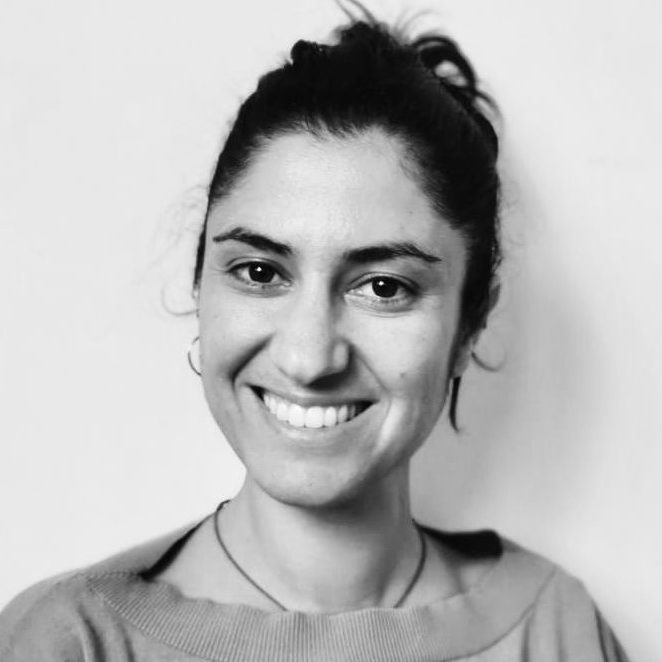
By Giorgia Zaetta – May. 19, 2021
G protein-coupled receptors (GPCRs), also known as heptahelical or 7-transmembrane receptors, are the largest family of membrane receptors. They are implicated in many human pathologies, and they represent an exceptionally important class of receptors to be studied, as they are crucial to key physiological functions from neurotransmission to cell growth to blood pressure regulation. A recent review showed that ~35% of approved drugs address GPCRs as targets (primarily small molecules and peptides), which makes this family of receptors one of, if not the most, valuable classes of drug targets in the human body.
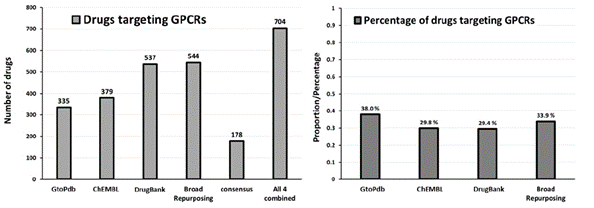
GPCRs: Problems and Limitations
Despite increasingly successes in experimentally solved GPCR crystallographic structures, those available represent only a small fraction of the total GPCRs (Katritch et al., 2013). There is therefore an important responsibility of the scientific community to maximize the effort of solving structures for the remaining receptors and complexes. Until that happens, the available structure-based computational help is valuable for probing GPCR’s ligand binding, however this rely on an X-ray crystal structure, which could be difficult to obtain for the GPCR of interest. With the little information available, usually each GPCR project involves numerous challenges, and requires different approaches to best model the interaction between the GPCR of interest and the specific ligands.
Solutions and Advantages of CADD
Although progresses in structure determination of GPCRs has made it possible to apply structure-based drug design (SBDD) methods to this pharmaceutically important target class, the availability and the quality of those available structures falls in a very narrow window. Moreover, most targets for which a structure has been solved have typically only one ligand co-crystallized, making it necessary to predict the binding mode of other compounds using computational techniques.
Some examples of alternative computational approaches to determining models of a GPCR 3D protein structures are loop prediction, homology models and machine learning. On one hand loop prediction is done with the GPCRs’ transmembrane domains fixed in their crystallographic positions, while the loops are computationally predicted in sequence, on the other hand the homology model (Krieger et. al., 2003) is performed using the structure arising from one known sequence of amino acids, and that knowledge of the structure A is used to predict a similar structure B. Although homology models rely on docking and SBDD, for each solved GPCR structure there are enough close homologues that can be modeled with relatively high accuracy.
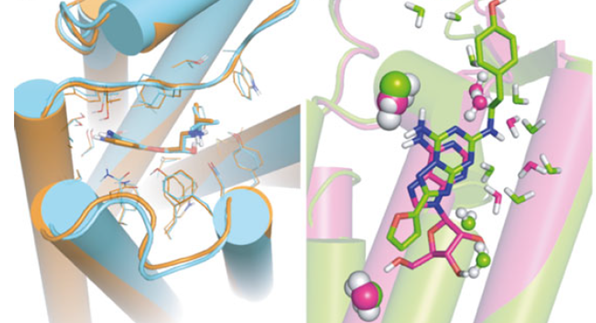
Ligand-based models are developed using the molecular representations of a reference molecule. These representations include the molecular physicochemical properties or molecular fingerprints of the given molecule (Lo et. al., 2018; Chen et. al., 2018). This is, to date, the best solution to GPCR above-mentioned limitations, having the possibility of exploring the ligands chemical space with no restrictions associated to the lack of structural information, as commonly happens when dealing with GPCRs. A recent study (Marawan et. al. 2021), also describes a machine learning model used for drug-repurposing against GPCRs, as a valid alternative to finding new drugs. In the study, a dataset of > 500K data points (with annotated data of > 160K unique ligands against > 250 GPCRs), was used to validate the ML) model, displaying an excellent performance.
We, at Pharmacelera, use AI and machine learning to train and improve our models to mine an unexplored chemical space and find hits with larger chemical diversity. In a case study on a GPCR set, we validated the capability of PharmScreen, our ligand-based software, to compute 3D molecular similarity based on hydrophobicity derived from the quantum mechanical version of the Miertus − Scrocco − Tomasi (MST) continuum model. The set of 21 GPCR targets, which only include active molecules with an alternative scaffold compared to the reference structure, were compiled. Outstanding results were obtained in the initial 5% of all the sets, with numerous hits found with a wide chemical diversity.
Conclusions and future perspectives
There will always be the urgency to develop better and faster computational models that can identify potential drug candidates against GPCRs. Even if this is supported by the recent advances in high performance computing, machine learning, advanced CADD tools and novel strategies to investigate new drugs, there are still needs to be met.
PharmScreen, our ligand-based software, using unique and superior 3D representation of molecules, is an alternative to traditional computational approaches. It overcomes the bias commonly associated with other ligand-based approaches, because the hits it identifies are independent from the ligand-receptor interaction of the active reference compound, and it is an excellent complement to structure-based approaches.
Do you want to find out how PharmScreen can help you run successfully your GPCR drug discovery projects? Contact us.
Bibliography
- “Loop prediction for a GPCR homology model: Algorithms and results”, Goldfeld, D. A. et. at., Proteins, 2013; 81, 214–228.
- “G Protein-Coupled Receptors as Targets for Approved Drugs: How Many Targets and How Many Drugs?”, Sriram, K. and Insel, P. A., Mol Pharmacol, 2018, 93, 251–258.
- “Advances in GPCR Modeling Evaluated by the GPCR Dock 2013 Assessment: Meeting New Challenges”, Kufareva I. et. al., Structure, 2014, 22, 1120–1139.
- “Docking and Virtual Screening Strategies for GPCR Drug Discovery”, Beuming, T. et. al., In: Filizola M. (eds) G Protein-Coupled Receptors in Drug Discovery. Methods in Molecular Biology, 2015, vol 1335.
- “GPCR_LigandClassify.py; a rigorous machine learning classifier for GPCR targeting compounds”, Marawan, A. et. al., Scientific Reports, 2021, 11, 9510.