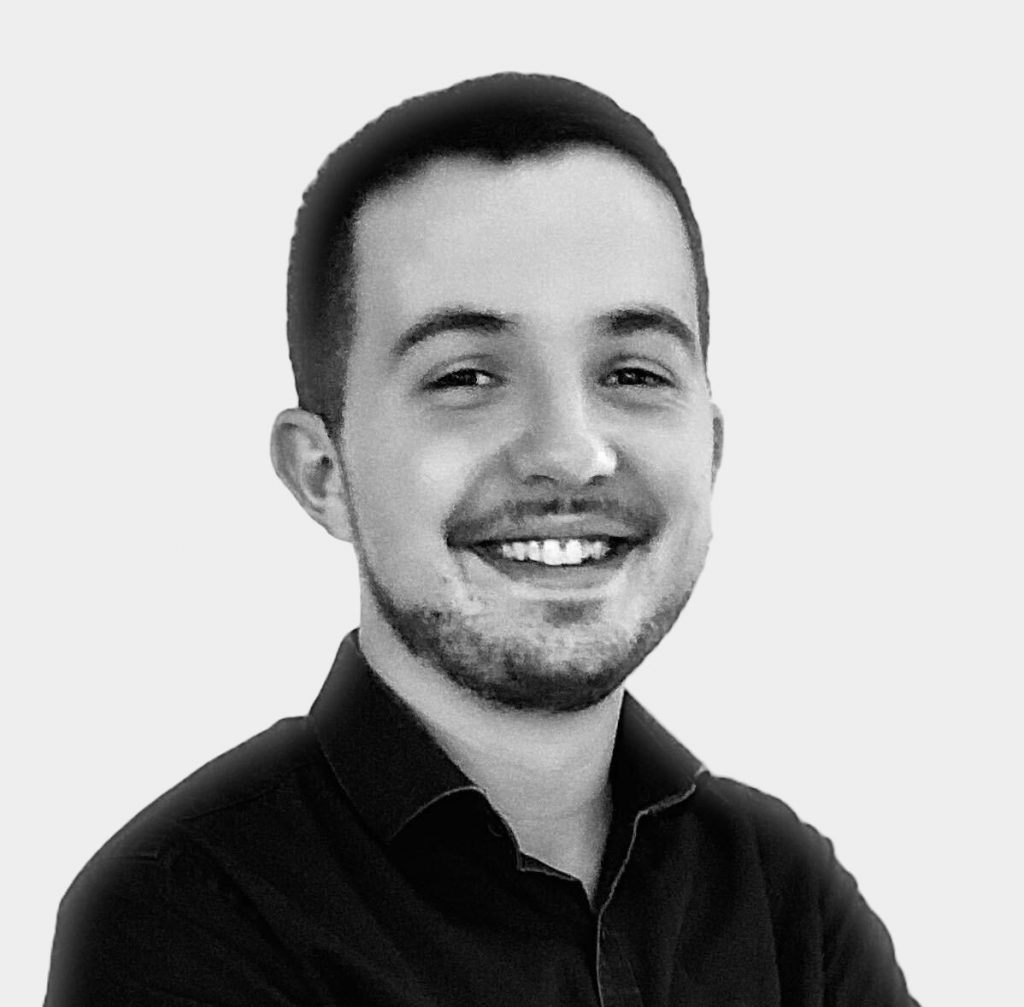
By Lluis Campos – Sep. 23, 2020
Molecular Docking is a key tool in Structure Based Drug Discovery (SBDD), as it aims to determine the predominant binding mode of a ligand to a target protein. The process requires 1) a three-dimensional structure of the target protein and 2) the generation of thousands of poses for the ligand, among which the program will try to find the best fit. To do that, Molecular Docking programs use a Scoring Function (SF) [1].
SFs are, hence, mathematical tools used in SBDD to evaluate protein-ligand interactions. Using advanced physics and complex theoretical models is not the aim of SFs, as it would suppose an unaffordable computational cost. Rather than that, SFs are based on approximations, allowing a higher calculation effectivity, i.e., a better balance between accuracy and speed [2].
The function of a Scoring Function
The ideal SF should be able to accomplish three main tasks. The first one is to identify the correct binding orientation of each ligand to the active site of the protein when choosing among all generated poses. The second is the selection of potential lead compounds against a target protein by screening a ligand library. And the third one is the prediction of the absolute binding affinity between the ligand and the target. It is generally assumed that current SFs perform satisfyingly well at pose prediction, while virtual screening and specially binding affinity prediction remain as major challenges.
What types of Scoring Functions can I find?
Current classifications [2] group SFs in four different types. Force field/Physics-based SFs, Empirical SFs and Knowledge-based potentials are usually referred as “classical SFs”, while in recent years a new group has been defined as Descriptor-based or Machine Learning-based SFs.
Physics-based SFs were the first ones to be developed and they are based on the physical atomic interactions between target and ligand. These are usually divided in Van der Waals forces, computed as Lennard-Jones potentials, and electrostatic forces, calculated using Coulomb’s Law. Since this approximation neglects both entropic and desolvation contributions, very often physics-based SFs incorporate additional terms to account for them. Examples of this kind of SFs are GoldScore in the docking software GOLD and SFs in Autodock 3 and 4.
Empirical SFs are probably the most intuitive approach among the four groups. They compute binding affinity by adding up a set of weighted energy terms. These terms represent energetic factors for the binding, such as hydrophobic effects, hydrogen bonds, halogen bonds, steric clashes, etc. Multiple Linear Regression is used to infere the weights from a training set of protein-ligand complexes with known binding affinities. Again, some of these terms are often devoted to account for the entropic and desolvation effects. Examples of empirical SFs are ChemScore from GOLD and GlideScore SP in Schrödinger’s Glide.
Knowledge-based SFs are based on the inverse Boltzmann statistic principle. This principle assumes that, in a training set of protein-ligand complexes, the frequency of a pair of atoms at a specific distance is directly proportional to the contribution of this pair to the binding energy. Hence, frequencies of different pairs are converted into distance-dependent potentials, and the binding affinity can be then computed as the sum of all pairwise potentials present in the complex.
The new trend: Machine learning-based Scoring Functions
Machine Learning methods are being currently applied to every single data-based field and Computer-Aided Drug Discovery is not an exception. Computational chemists are taking advantage of the increasing amount of experimental data that is available nowadays to build models that can achieve better results than the more classical SFs above described. Far from what has been exposed for the latter, where functional forms differed in specific terms but had a common scaffold, a myriad of different functional forms can be obtained depending on the algorithm chosen; from Support Vector Machines and Random Forest to the more complex and opaque Deep Neural Networks.
Functional forms of Machine learning-based SFs, also called descriptor-based by some reviewers [2], are very similar compared to empirical SFs. However, there are three major reasons to group them in another category. First, whereas classical SFs have linear functional forms, descriptor-based SFs have different functional forms depending on the machine learning algorithm employed. Second, the number of terms tends to be extremely larger in descriptor-based SF; while it is rare to find empirical SFs with more than ten weighted terms, here hundreds of different descriptors can be exploited. And third, while individual terms in an empirical SF have an easily interpretable physical meaning, this is not necessarily the case for descriptor-based SFs, which might operate as a “black box”.
The greatest asset of this kind of SFs is also the major weakness of classical models, i.e., binding affinity prediction. The predictive power of a SF is often measured as the Pearson correlation coefficient (Rp) between the experimental binding energy and the one predicted by the model. Two conclusions can be extracted from the table below [3]. The first one is that in descriptor-based SF largely outperform the classical approaches, even the ones with better scores. And the second is that no new relevant classical SFs have been developed since the machine learning wave raised.
Furthermore, it has been demonstrated that the predictive capacity of descriptor-based SFs tends to be improved when larger training sets are provided. In this sense, many databases are now publicly available with loads of data about ligand-protein affinities. Probably the most popular among them is PDBbind, which is updated on an annual basis, and currently stores almost 13,000 carefully curated biomolecular complexes, from which around 10,000 are protein-ligand complexes.
The future of Scoring Functions
From the exposed above, it seems clear that machine learning is going to play an important role on the development of the state-of-the-art molecular docking software of the future. For now, many studies have taken profit of the fact that descriptor-based SFs are orthogonal to classical ones, and they are using them as rescoring functions to improve the results obtained by the latter. On the other hand, it is still unclear how the size and content of the training set can affect their performance, since in many cases it is strongly dependent on the target protein family.
Meanwhile, other possibilities for the development of new ways of assessing protein-ligand complementarity remain unexplored. To our knowledge, only one study has been published on the use of Molecular Interaction Fields (MIF) to predict protein-ligand interaction [4]. The results are promising and open the door for future work on the field. Pharmacelera’s hydrophobic MIFs have shown outstanding results applied to ligand-based virtual screening campaigns, but they are still to be used on structure-based approaches.
So, what do you think? Are the Scoring Functions of the future going to be fully machine-learning driven? Will they rather be a perfect complement for classic SFs? Or, alternatively, are we going to find better docking protocols without the need of these complex algorithms?
References
[1] J. Li, A. Fu, and L. Zhang, “An Overview of Scoring Functions Used for Protein–Ligand Interactions in Molecular Docking,” Interdiscip. Sci. Comput. Life Sci., vol. 11, no. 2, pp. 320–328, 2019.
[2] J. Liu and R. Wang, “Classification of current scoring functions,” J. Chem. Inf. Model., vol. 55, no. 3, pp. 475–482, 2015.
[3] H. Li, K. H. Sze, G. Lu, and P. J. Ballester, “Machine-learning scoring functions for structure-based drug lead optimization,” Wiley Interdiscip. Rev. Comput. Mol. Sci., no. November 2019, pp. 1–20, 2020.
[4] D. Hayakawa, N. Sawada, Y. Watanabe, and H. Gouda, “A molecular interaction field describing nonconventional intermolecular interactions and its application to protein–ligand interaction prediction,” J. Mol. Graph. Model., vol. 96, p. 107515, 2020.