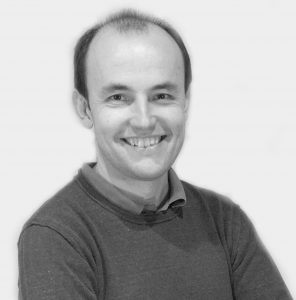
Enric Herrero
May 30th, 2023
Ligand-based virtual screening is a well-known technique to find hits in the early stages of a drug discovery program. In ligand-based tools, similarity between compounds is calculated to find which ones are more similar to a known active reference structure with the assumption they will interact similarly. In these tools molecular descriptors play an important role in the overall performance and speed of the tool, being 2D fingerprints one of the most used due to its compactness and reduced computational cost.
However, molecules have multiple properties that are dependent on the 3D geometry in space. Therefore, ignoring this information might affect the virtual screening performance. The value of 3D descriptors has been studied for many years in order to understand if the additional computational cost is justified. These studies1 have shown that 3D methods are better at finding compounds with lower structural similarity and, therefore, better finding diverse scaffolds.
In this post we are going to show an example that highlights the value of 3D descriptors in traditional libraries and also when exploring huge chemical spaces with billions of molecules with smart enumeration.

We have performed an experiment using a dataset of 1M molecules including known active structures (Hits) of the Histamine receptor (H1) and used CHEMBL1628227 as reference structure for the screening. In this post we are going to see if we can retrieve a hit with a different scaffold (CHEMBL16694007). We have used two types of descriptors to compare 2D and 3D methods: The Morgan fingerprint2, one of the most used 2D representations and the 3D hydrophobic profile3.

If we calculate the similarity of the reference against all the library with both descriptors, we can see that using 2D descriptors the hit of interest obtains a very low Tanimoto (Tn) score and is not selected among the first 0.1% of the molecules (first 1000). On the other hand, we can clearly see that the two molecules, despite having a different structure, have a very similar hydrophobic profile. This similarity is also translated into the ranking prioritizing the hit among the top 0.1% and highlighting the value of this type of descriptors.
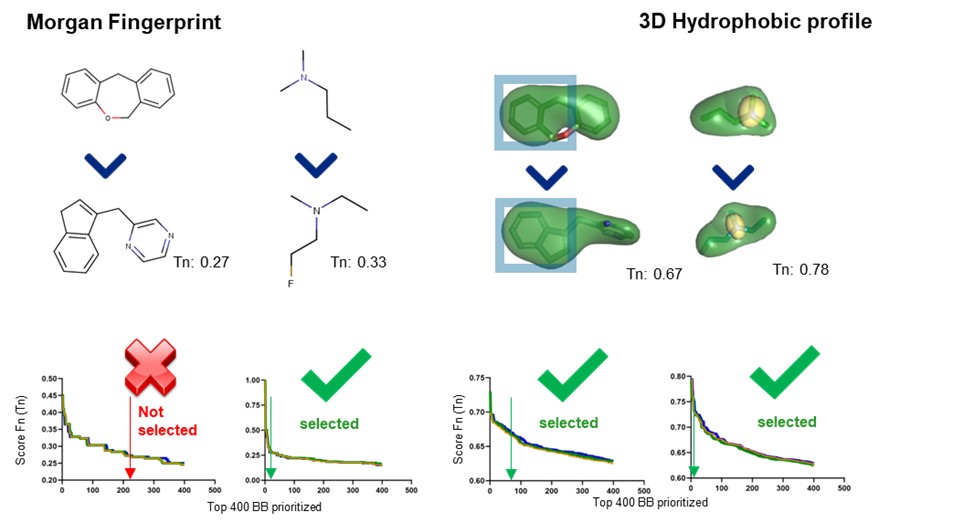
In order to see how this comparison stands when screening huge chemical libraries of billions of molecules, we have used the smart enumeration approach for the same example. In this case, instead of comparing the whole molecule against the molecule library we have compared each reference fragment against a building block library containing the hit fragments.
It can be seen in Figure 3 how in this case using the 2D descriptor we are able to select one of the two hit fragments. As expected, the selected hit fragment corresponds to the one that shares more structural similarity with the reference fragment. Since we are not able to select both hit fragments, we would not be able to identify this hit. Once again, in this case we can also see that the hydrophobic profile of the fragments is similar, and we would be able to find the hit in a virtual screening.
Overall, we have seen that the usage of 3D descriptors enables finding alternative scaffolds that 2D methods would not detect. In the advent of huge chemical libraries with billions of compounds, innovative solutions are needed to explore them. We have shown that in this new era 3D descriptors will still play an important role and using them will enable finding more chemical diversity.
If you want to learn more about how to use the 3D hydrophobic profile in ligand based virtual screening of huge chemical libraries, contact us.
References
[1] Hawkins, P. C. D.; Skillman, A. G.; Nicholls, A. Comparison of Shape-Matching and Docking as Virtual Screening Tools. J Med Chem 2007, 50, 74–82
[2] Rogers, D.; Hahn, M. “Extended-Connectivity Fingerprints.” J. Chem. Inf. and Model. 50:742-54 (2010).
[3] Vázquez, J.; Deplano, A.; Herrero, A.; Ginex, T.; Gibert, E.; Rabal, O.; Oyarzabal, J.; Herrero, E. and Luque, F.J. Journal of Chemical Information and Modeling 2018 58 (8), 1596-1609